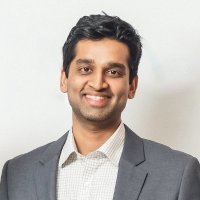
Karthik Narasimhan
@karthik_r_n
Associate Professor @PrincetonCS, Head of Research @SierraPlatform. Previously @OpenAI, PhD @MIT_CSAIL, BTech @iitmadras
ID: 3272351166
http://www.karthiknarasimhan.com/ 09-07-2015 01:28:42
259 Tweet
3,3K Followers
456 Following

SWE-bench Lite is a smaller & slightly easier *subset* of SWE-bench, with 23 dev / 300 test examples (full SWE-bench is 225 dev / 2,294 test). We hopes this makes SWE-bench evals easier. Special thanks to Jiayi Geng for making this happen. Download here: swebench.com/lite
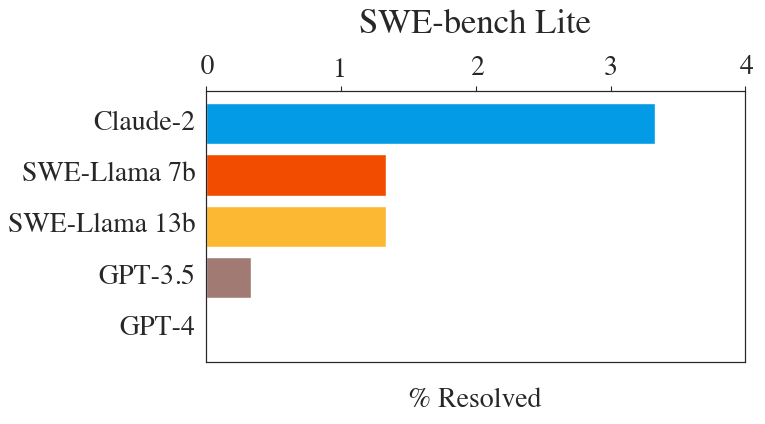
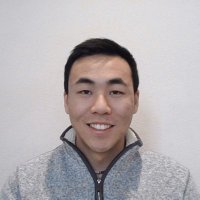

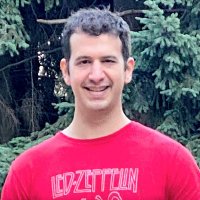
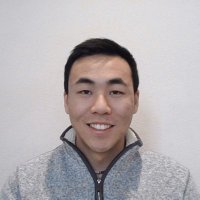
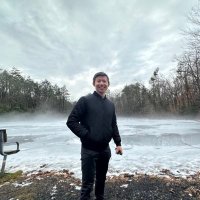
Our visualizer for our preprint, “Can Language Models Solve Olympiad Programming” is live. See the per-problem performance of models on USACO + more! Link here: princeton-nlp.github.io/USACOBench/ Ty again to my collaborators: Michael Tang Shunyu Yao Karthik Narasimhan
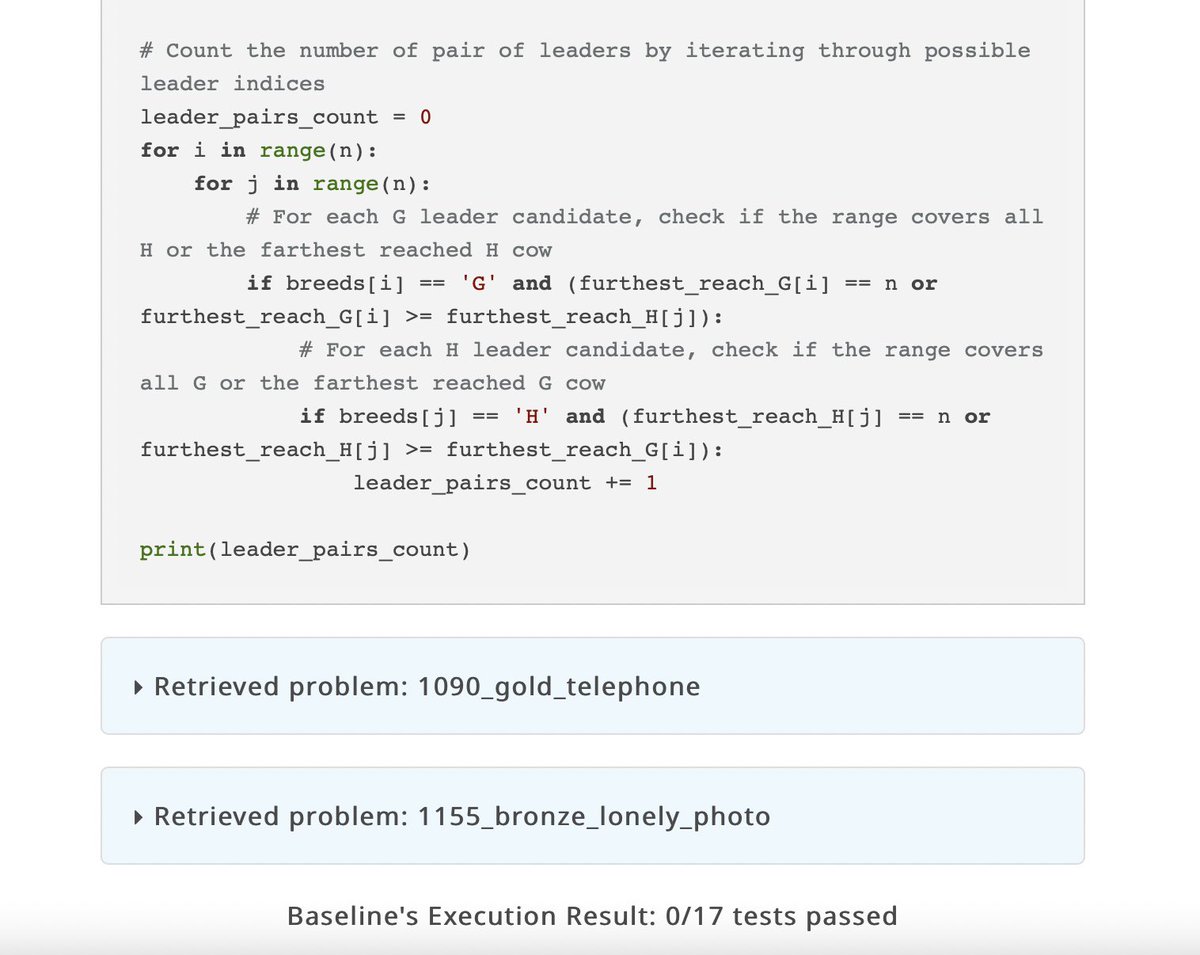

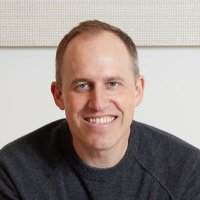
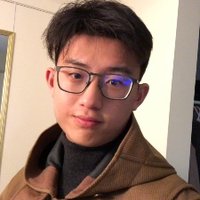
Excited to share what I did Sierra with Noah Shinn pedram and Karthik Narasimhan ! 𝜏-bench evaluates critical agent capabilities omitted by current benchmarks: robustness, complex rule following, and human interaction skills. Try it out!