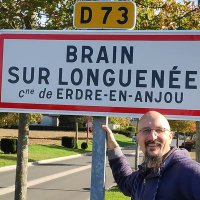
7. The seventh chapter critically examines the standardization of preprocessing in neuroimaging. osf.io/42bsu #Neuroimaging #fmriprep #BIDS #mriqc #reproducibility Oscar Esteban
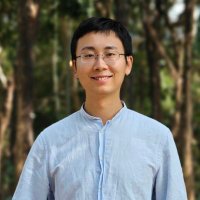
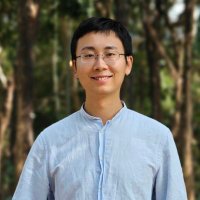
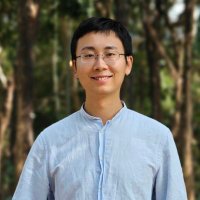
πExcited to share our #preprint on DeepPrep: a high-speed, scalable preprocessing pipeline for s/fMRI, empowered by SOTA #deeplearning algorithms. What takes #fMRIPrep 7 hrs, DeepPrep achieves in just 40 mins! Dive into the detailsπ§΅ Hesheng Liu Ted Satterthwaite shorturl.at/eBEP8
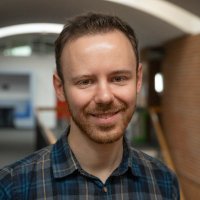
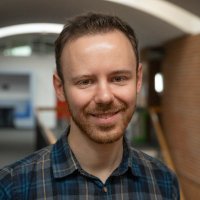
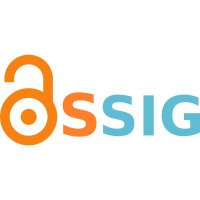
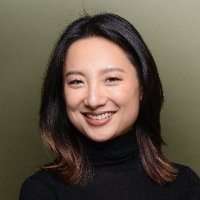
Attending Russ Poldrack's live talk! Looking forward to gaining insights from the #fmri preprocessing pipeline #fMRIPrep, applying some ideas with our EEGManyPipelines project, especially exploring the "glass box" visualization! #OHBMBrainhack #workflows
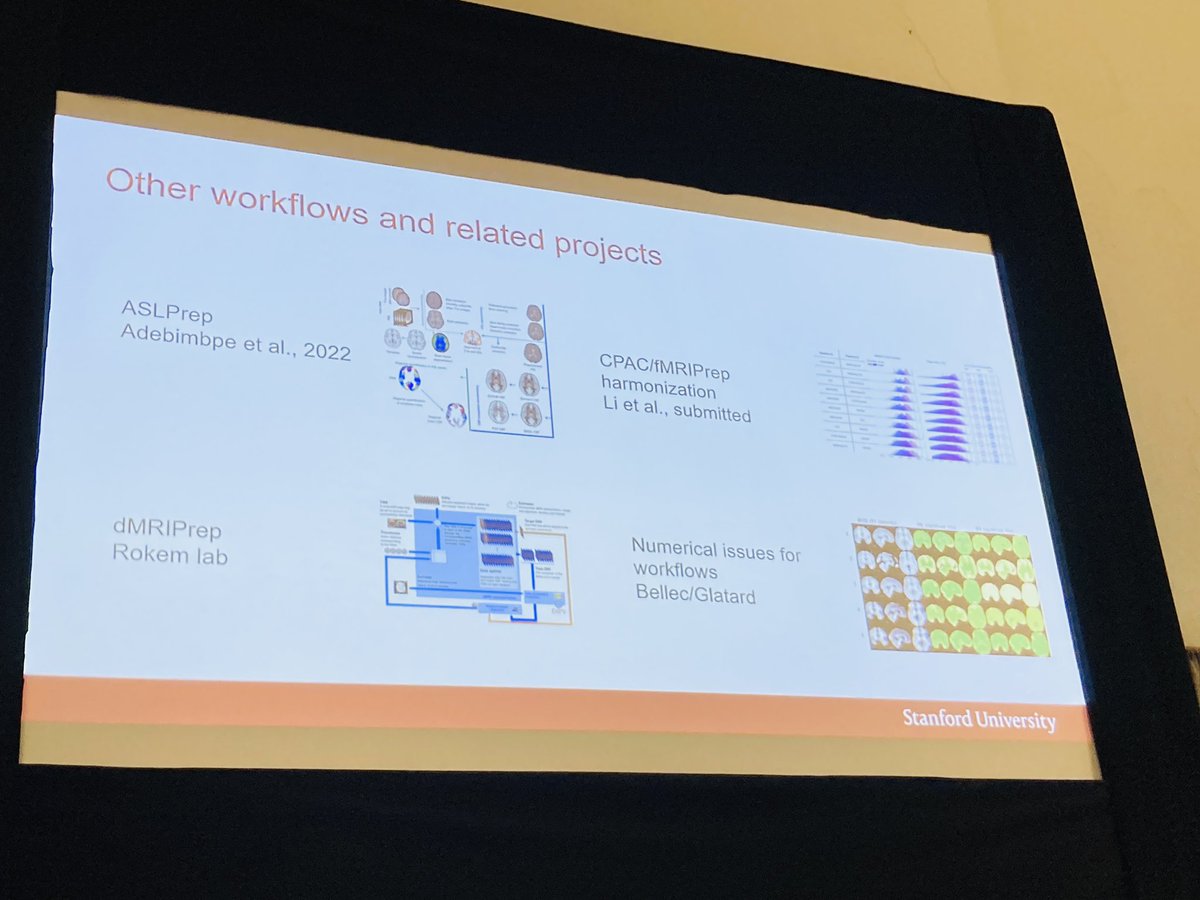
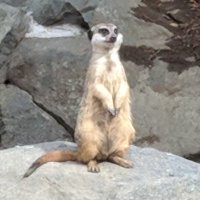
We apply this reproducible benchmark to investigate the robustness of the conclusions across two OpenNeuro.org datasets and two LTS versions of #fMRIPrep. 5/8
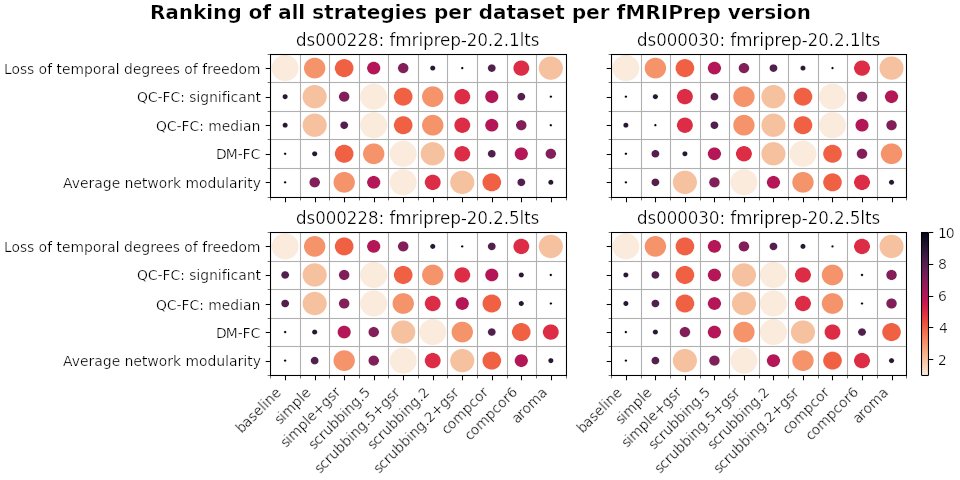
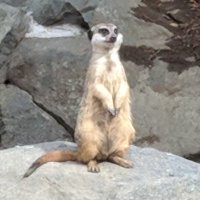
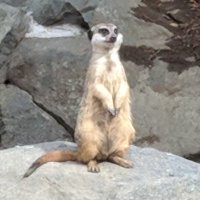
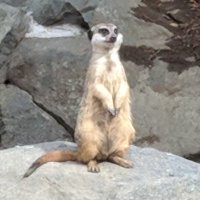
Our work βA reproducible benchmark of resting-state #fMRI denoising strategies using #fMRIPrep and Nilearnβ is now officially on the reproducible preprint service CONP / PCNO and bioRxiv Neuroscience π neurolibre.org/papers/10.5545β¦ 1/8
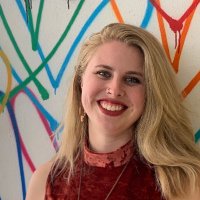
Anyone collecting #multiecho #MRI data (including phase reversed images) and using #fMRIPrep? How are you setting up the AP/PA pairs in distortion correction? OHBM Trainees
Thomas Yeo We encourage users to copy #fMRIPrep's boilerplate verbatim to minimize errors in reporting. The boilerplate is released with public domain licensing terms to facilitate that.
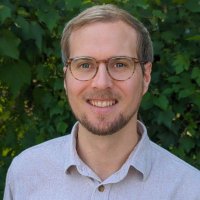
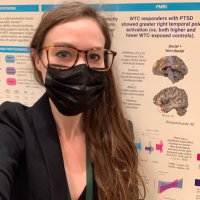
Without Twitter, I never would have applied for ππ’ππ π ππππππ's reproducible neuroscience workshop or Neurohackademy... Since then, my PhD and now postdoc work involves using or helping others use #fMRIPREP on a weekly basis, & I recently worked on a manuscript with Oscar Esteban π₯²