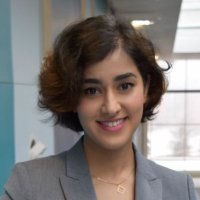
Generative Models of Brain Dynamics – A #review
🎁 After a year of work, our #Xmas present is freshly out: arxiv.org/abs/2112.12147 🎄
Get a bird's eye view with the synthesis of >200 refs at the intersection of #ML , #DynamicalSystems , and #Neuroscience !
A thread…🧵 (1/5)
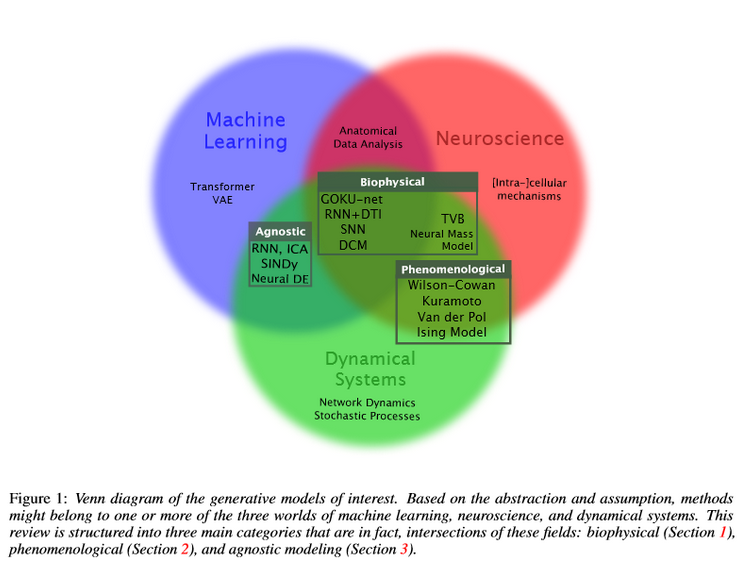
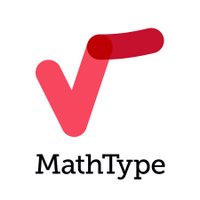
In the 60s, Edward N. Lorenz stumbled upon the concept of chaos. When using early computers to predict weather he realized that small differences in the initial conditions of the system had a huge impact long term: the Butterfly Effect #DynamicalSystems #ChaosTheory #MathType
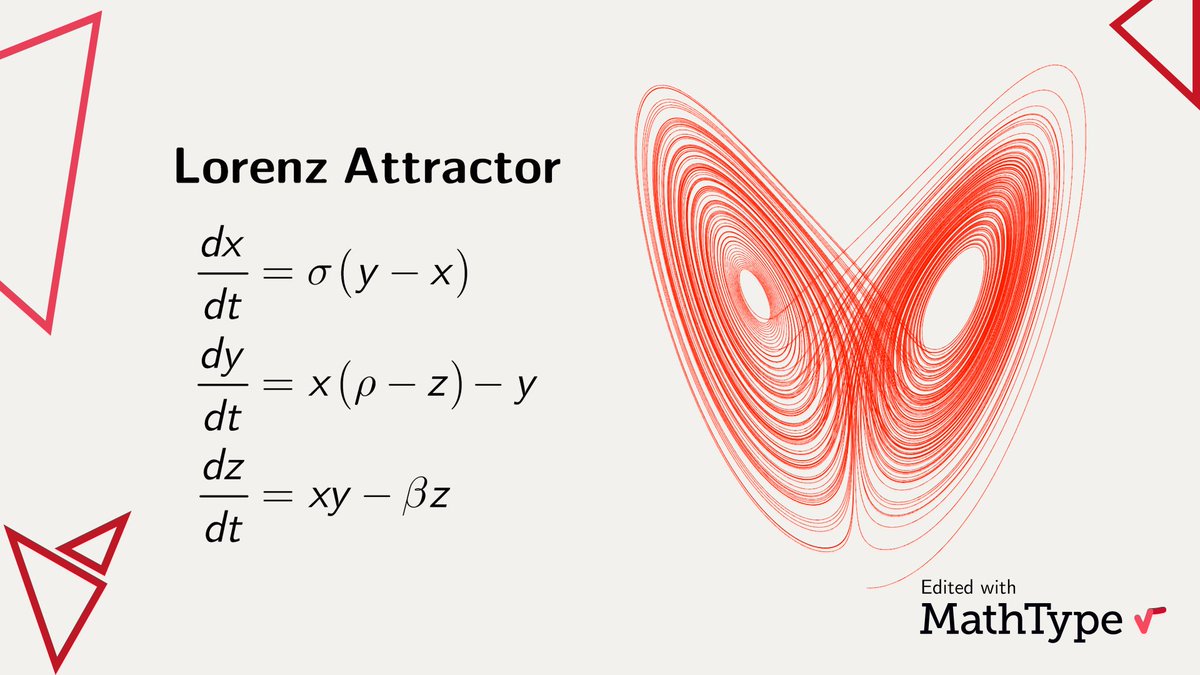
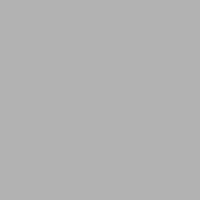
Fishing up consumer-resource dynamics this week with the Rosenzweig-MacArthur model:
#rstats
#dataviz
#DynamicalSystems
#PredatorPrey
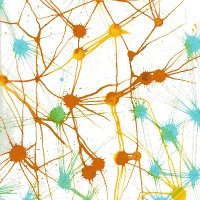
In science we often access the same system via many diff. data channels simultaneously, e.g. neural activity and behavior in #neuroscience . #ML
Here we develop a general framework for nonlinear #DynamicalSystems reconstruction based on *multi-modal* RNNs: arxiv.org/abs/2111.02922
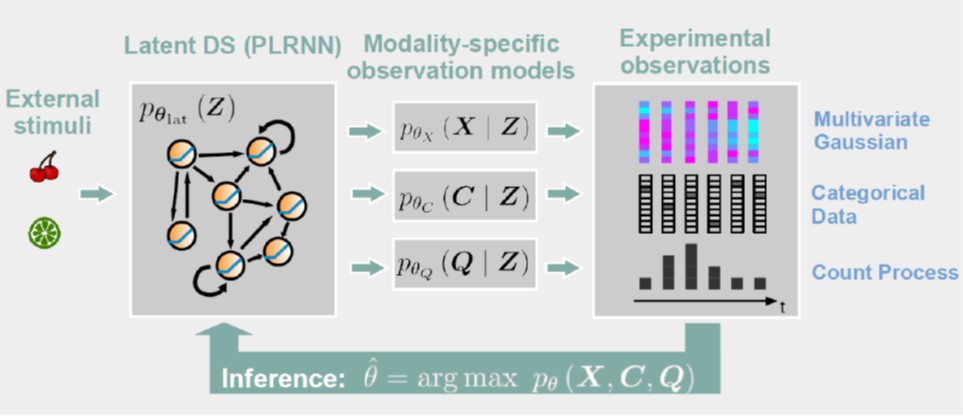
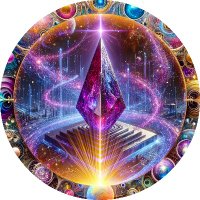
Deep Learning of Conjugate Mappings
Bramburger et al.: arxiv.org/abs/2104.01874
#DeepLearning #DynamicalSystems #MachineLearning
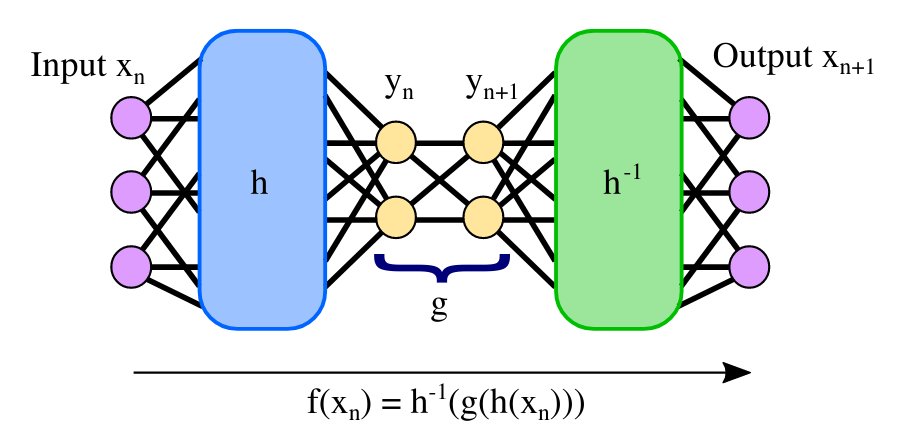
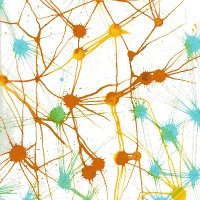
Our Perspective on reconstructing computat. system dynamics from neural data finally out in Nature Rev Neurosci!
nature.com/articles/s4158…
We survey generative models that can be trained on time series to mimic the behavior of the neural substrate.
#AI #neuroscience #DynamicalSystems
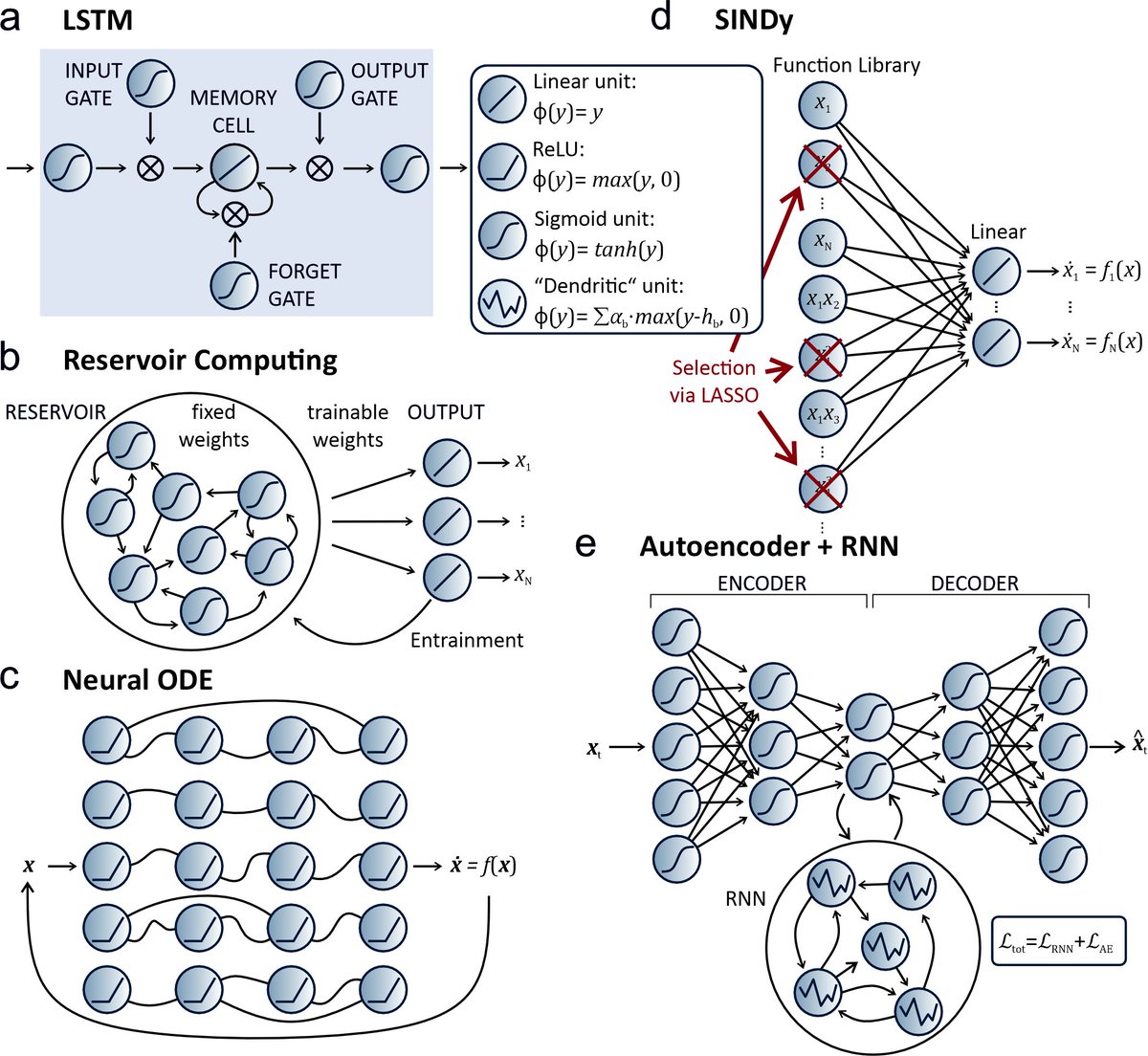
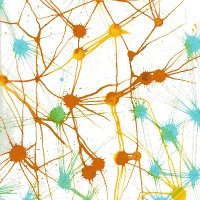
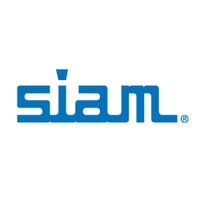
Innovations in #MachineLearning —particularly #DeepLearning —have yielded new insights into the connection between #DynamicalSystems and #DataScience . In SIAM News, Qianxiao Li and Weinan E introduce some recent lines of work at this intersection. Read more: sinews.siam.org/Details-Page/m…
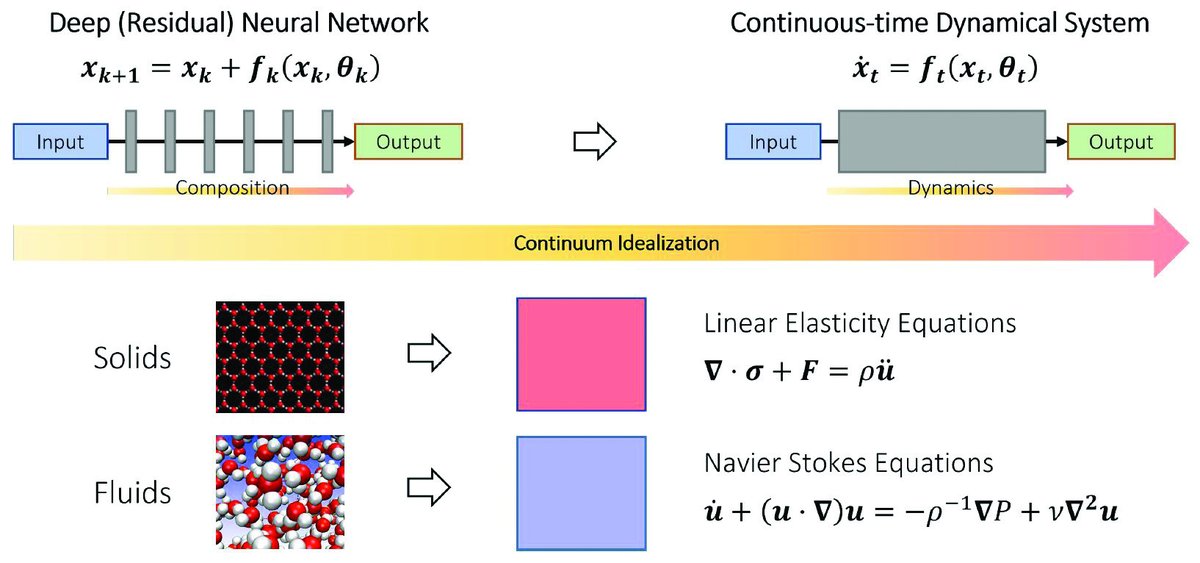

Analog computer is up and running Lancaster University Phonetics Lab, solving some differential equations. Will post examples soon!
#dynamicalsystems #phonetics #analogcomputer
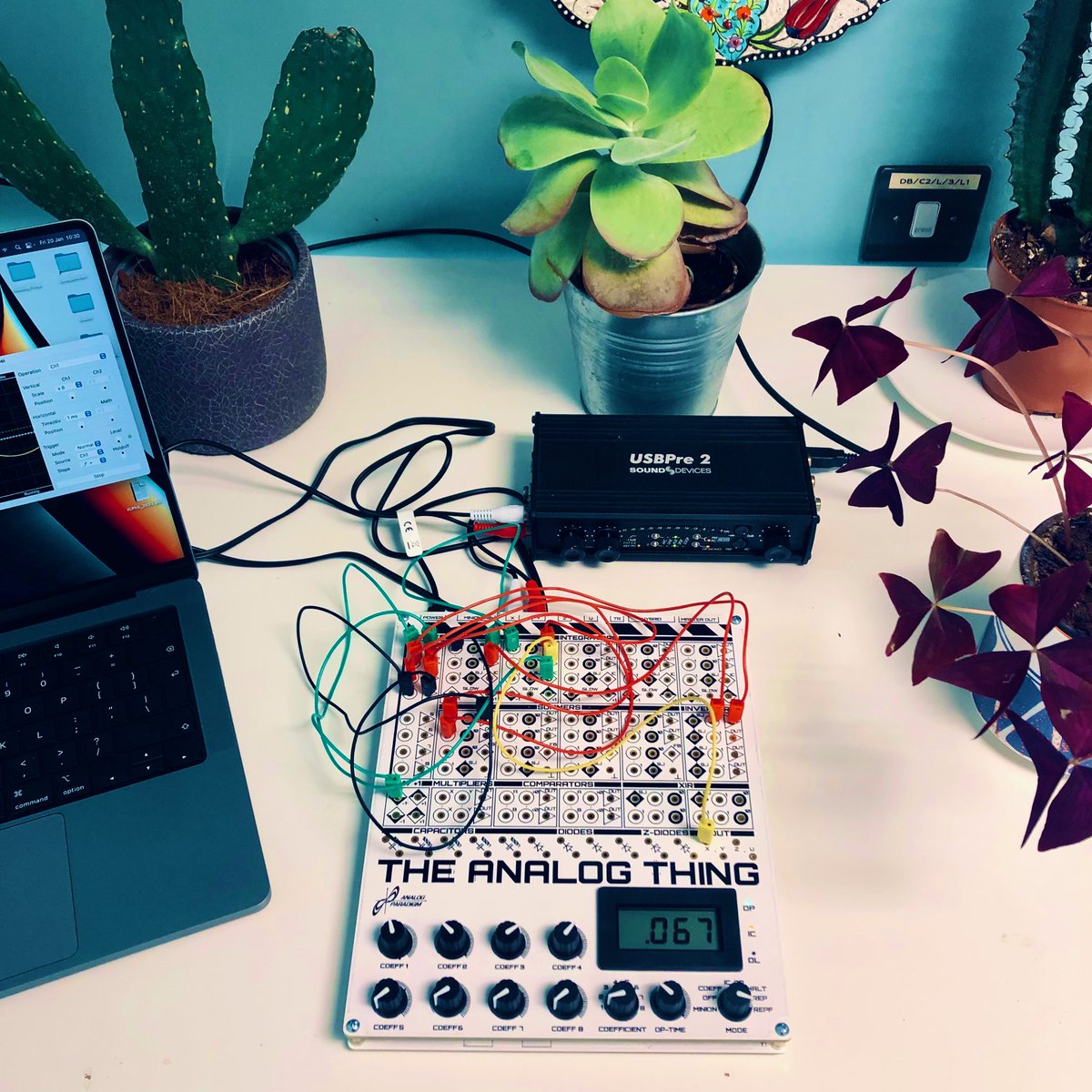
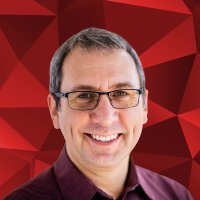
Is the finite-time Lyapunov exponent field a Koopman eigenfunction?
📢New open-access paper with Erik Bollt ➡️mdpi.com/1332916
#DynamicalSystems #FluidDynamics SIAM Activity Group on Dynamical Systems
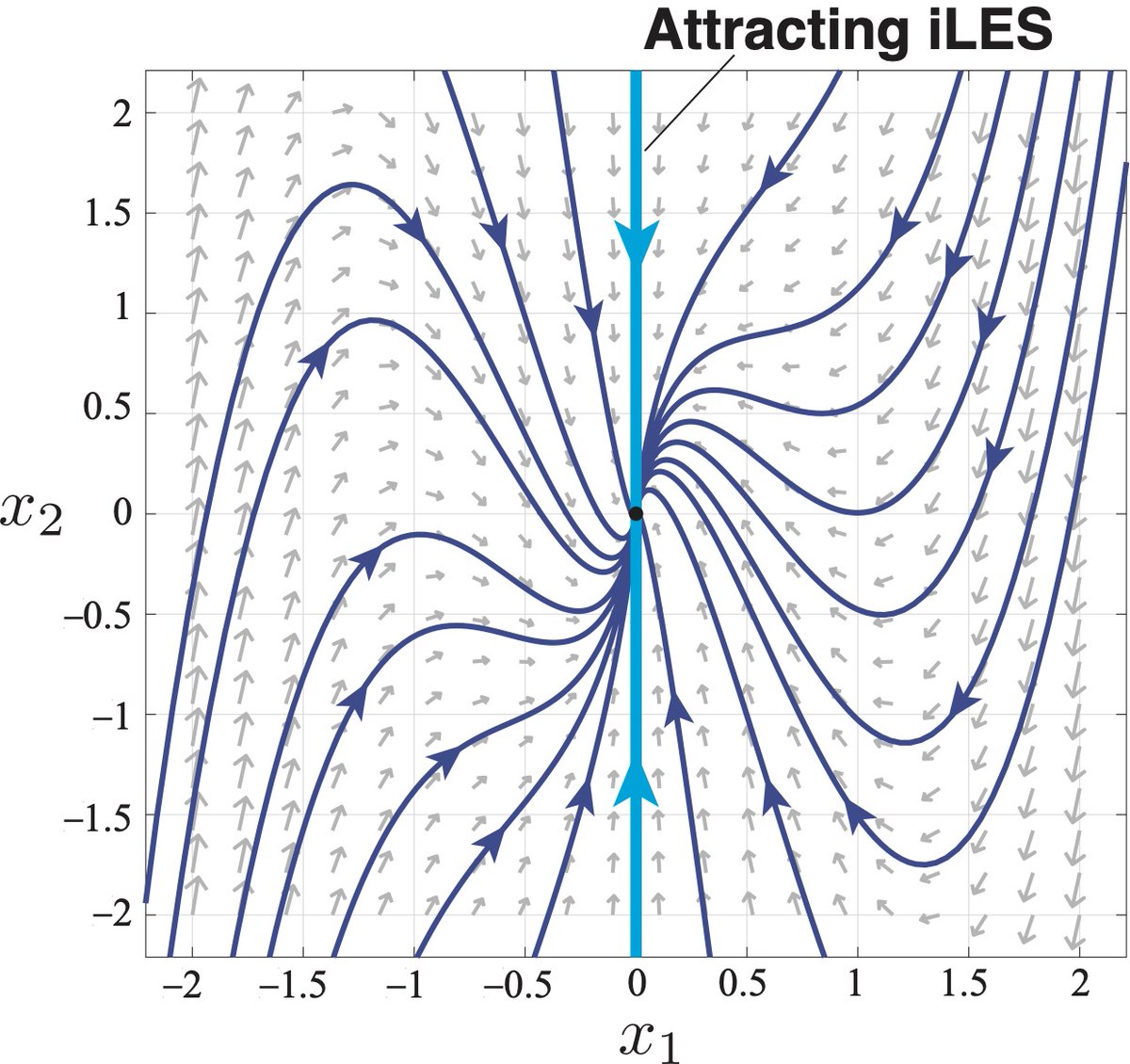
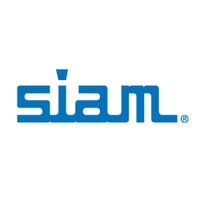
The dynamic mode decomposition is a powerful technique for the discovery of #DynamicalSystems from high-dimensional #data . In SIAM News, J. Nathan Kutz, Steven Brunton, Bing Wen Brunton, and Joshua L. Proctor present an excerpt from their book on DMD. Read more: sinews.siam.org/Details-Page/d…
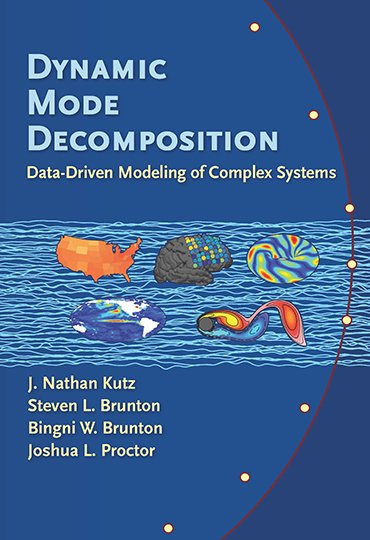
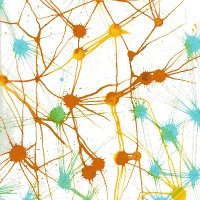
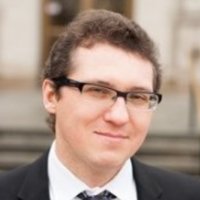
Our work on Converse Symmetry Breaking is about to appear in Nature Physics.
Out on Monday, January 20!
I’ll be talking about it at 𝙲𝚘𝚖𝚙𝚕𝚎𝙽𝚎𝚝 𝟸𝟶𝟸𝟺 2020.
Teaser below.
#dynamicalsystems #networkscience
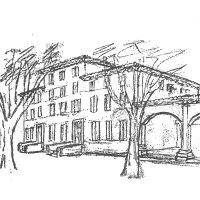
CIRM 🆕🎬Multidimensional symbolic dynamics and lattice models of quasicrystals
Kucherenko, Tamara (2024). Phase transitions on one-dimensional symbolic systems. CIRM. Audiovisual resource.
dx.doi.org/10.24350/CIRM.…
CIRM #Maths #conference #Video #DynamicalSystems
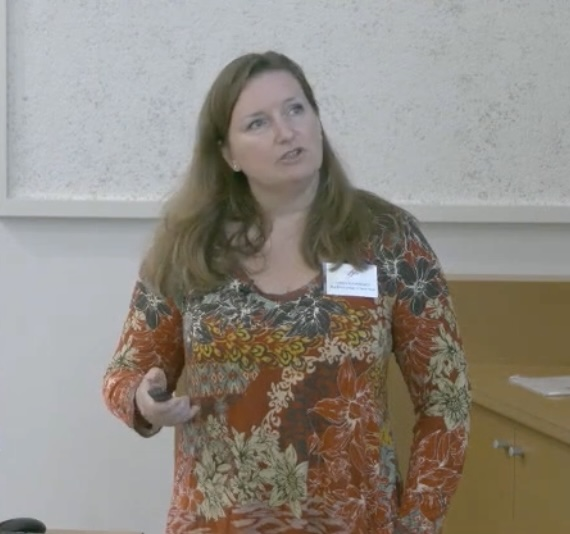
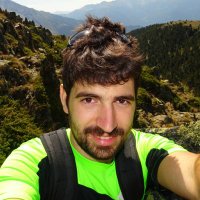
After years of work, the day is finally approaching! This Thursay at 9:00 CET, I will defend my thesis!
Topic: #Terraforming Earth's Ecosystems #DynamicalSystems #Ecology #SyntheticBiology #Anthropocene
Supervised by Ricard Solé, Josep Sardanyés & Nuria Conde-Pueyo
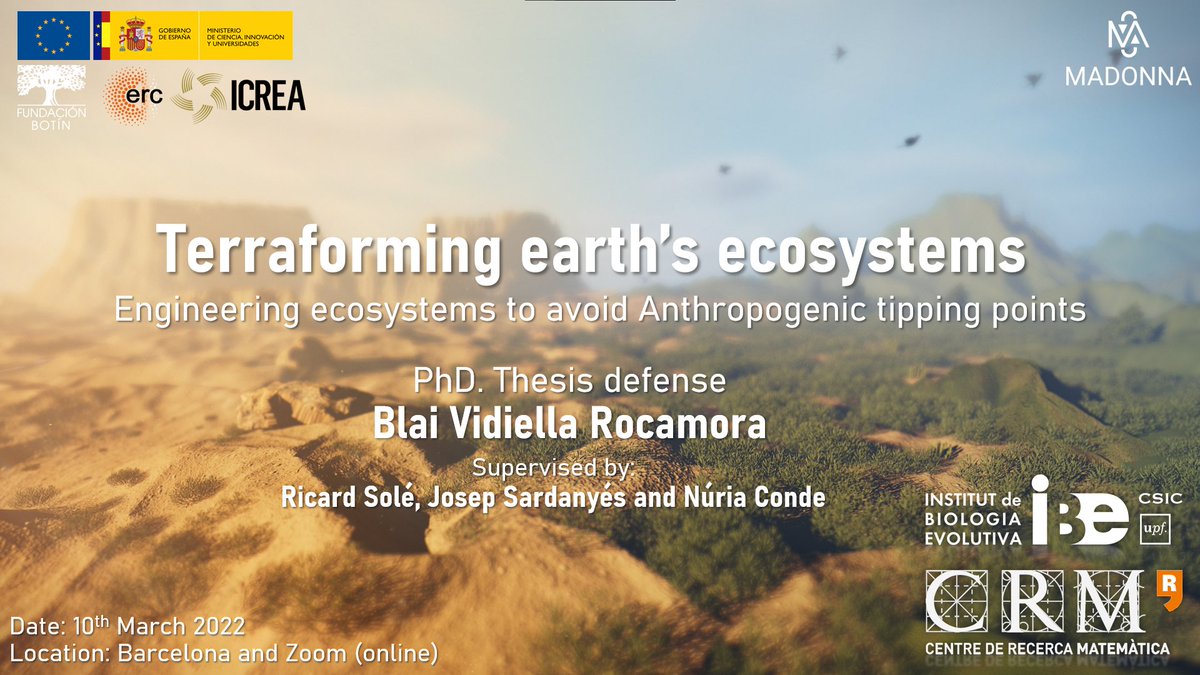
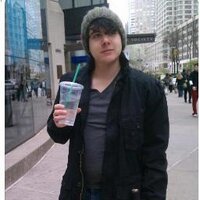
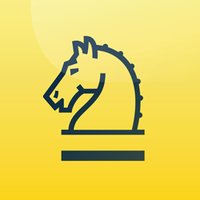
Recently accepted by #InventionesMathematicae , 'Persistence of heterodimensional cycles' by Dongchen Li and Dmitry Turaev Imperial College London
#DynamicalSystems
🔓 #OpenAccess
link.springer.com/article/10.100…
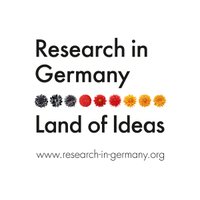
Take a look: the research group on 'Multiscale and Stochastic Dynamics' at TU München sees to fill a #PhD position in #AppliedMathematics ow.ly/vwdJ50AsLPL #PhD Germany #DynamicalSystems #StochasticProcesses #ScientificComputing [image: © Astrid Eckert / TUM]
![Research in Germany - Initiative of the BMBF (@ResearchGermany) on Twitter photo 2020-07-16 02:35:03 Take a look: the research group on 'Multiscale and Stochastic Dynamics' at @TU_Muenchen sees to fill a #PhD position in #AppliedMathematics ow.ly/vwdJ50AsLPL #PhDGermany #DynamicalSystems #StochasticProcesses #ScientificComputing [image: © Astrid Eckert / TUM] Take a look: the research group on 'Multiscale and Stochastic Dynamics' at @TU_Muenchen sees to fill a #PhD position in #AppliedMathematics ow.ly/vwdJ50AsLPL #PhDGermany #DynamicalSystems #StochasticProcesses #ScientificComputing [image: © Astrid Eckert / TUM]](https://pbs.twimg.com/media/EdA7KOVWsAAsRdc.jpg)
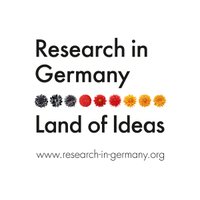
The research group on 'Multiscale and Stochastic Dynamics' at TU München sees to fill a #PhD position in #AppliedMathematics - Check it out! ow.ly/6qTs50AsM5F #PhD Germany #DynamicalSystems #StochasticProcesses #ScientificComputing [image: © Astrid Eckert / TUM]
![Research in Germany - Initiative of the BMBF (@ResearchGermany) on Twitter photo 2020-07-21 01:25:03 The research group on 'Multiscale and Stochastic Dynamics' at @TU_Muenchen sees to fill a #PhD position in #AppliedMathematics - Check it out! ow.ly/6qTs50AsM5F #PhDGermany #DynamicalSystems #StochasticProcesses #ScientificComputing [image: © Astrid Eckert / TUM] The research group on 'Multiscale and Stochastic Dynamics' at @TU_Muenchen sees to fill a #PhD position in #AppliedMathematics - Check it out! ow.ly/6qTs50AsM5F #PhDGermany #DynamicalSystems #StochasticProcesses #ScientificComputing [image: © Astrid Eckert / TUM]](https://pbs.twimg.com/media/EdabFn3XgAAPba-.jpg)
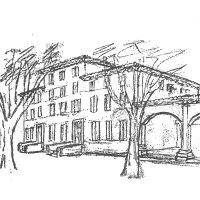
🆕🎬Multidimensional symbolic dynamics and lattice models of quasicrystals
Kra, Bryna (2024). Symmetries in symbolic dynamics. CIRM. Audiovisual resource. dx.doi.org/10.24350/CIRM.…
CIRM #Maths #conference #Video #DynamicalSystems
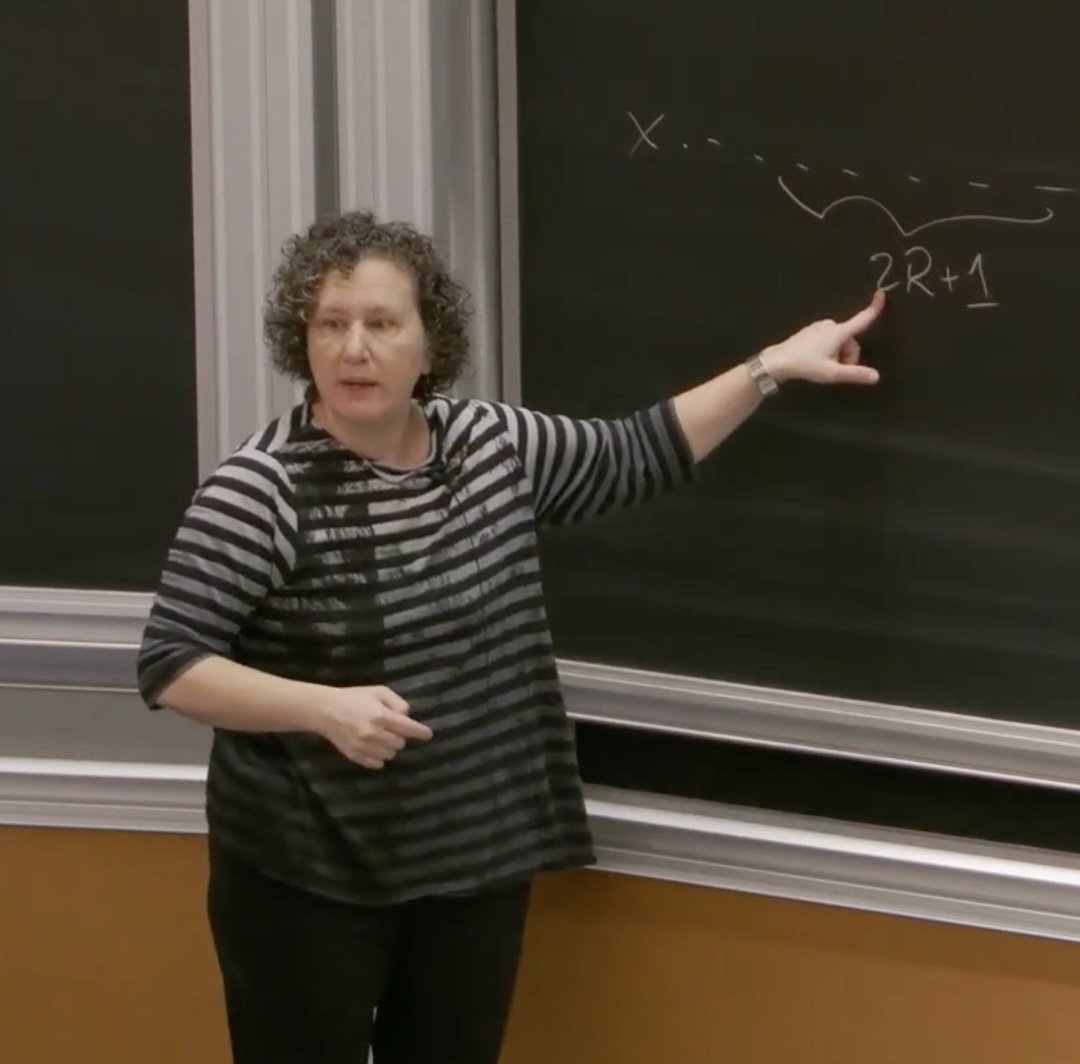