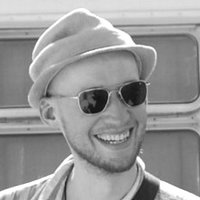
Maximilian Nickel
@mnick
Senior Random Hypothesis Generator at FAIR, Meta | AI ∩ Complex Systems ∩ Society | Program Chair ICLR'23 | Former { MIT, IIT, LMU, Siemens }
ID: 17823114
http://maxn.io 03-12-2008 00:14:13
470 Tweet
1,1K Followers
527 Following