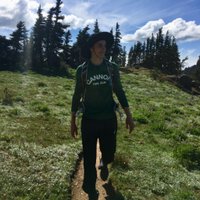
Eric
@ericmitchellai
I like AI & music. Working on making LLMs easier & safer to use. Final year PhD student at Stanford advised by Chelsea Finn & Chris Manning.
ID:942749627627065344
https://ericmitchell.ai 18-12-2017 13:33:21
569 Tweets
3,6K Followers
487 Following

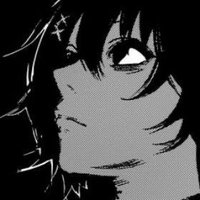
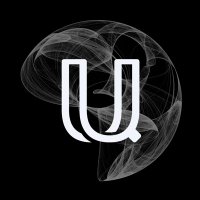
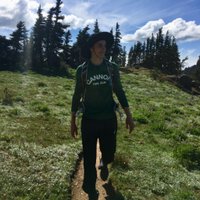

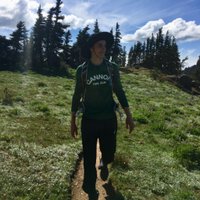
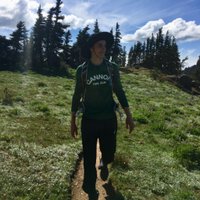
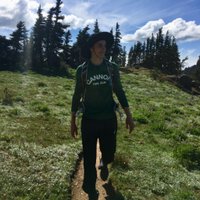
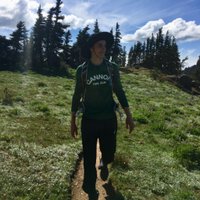
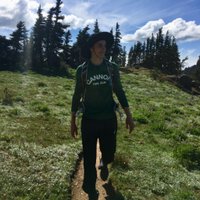
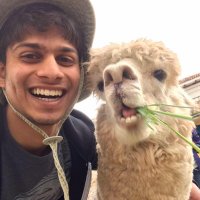
โOne needs to learn to love and enjoy the little things in life. One also needs to discover oneโs true calling and then should do everything to pursue the selected path,โ - wise words Archit Sharma
tribuneindia.com/news/amritsar/โฆ
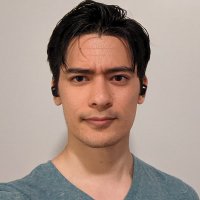
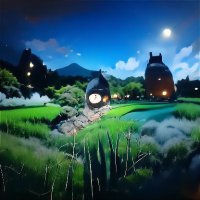