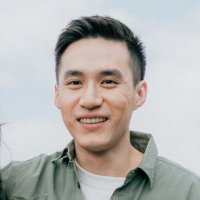
Guan-Horng Liu
@guanhorng_liu
Research Scientist @MetaAI (FAIR NYC) • Schrödinger Bridge, diffusion, flow, stochastic optimal control • prev ML PhD @GeorgiaTech 🚀
ID: 908526953061392385
http://ghliu.github.io 15-09-2017 03:04:40
202 Tweet
749 Followers
324 Following
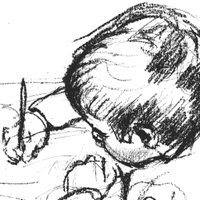
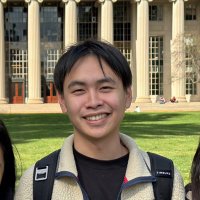
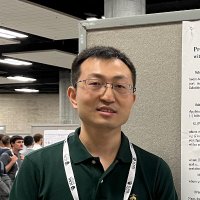

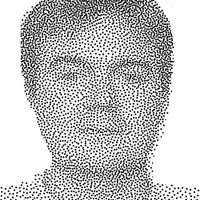
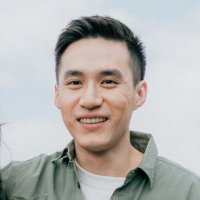
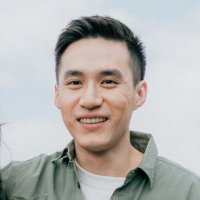
🚨🚨 Deadline of #SPIGM workshop at #ICML2024 is now #May27 #AoE, with page limit relaxing from #4to8 pages. For any questions, join our Slack (faster) or send us emails (slower) Slack invite link: see spigmworkshop2024.github.io) ✉️: [email protected]
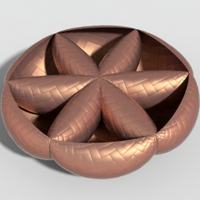
Interested in learning about differential geometry and its connection to geometric computing? All material from the Carnegie Mellon University course on #DiscreteDifferentialGeometry has been collected in a new webpage (videos, code, exercises, etc.). Check it out! geometry.cs.cmu.edu/ddg

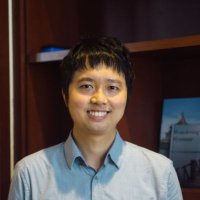
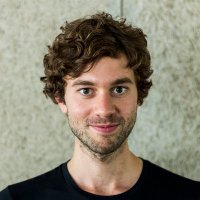
I gave a talk on our latest work on the connections between dynamical systems, PDEs, control and path space measures for sampling from densities at the The Fields Institute in Toronto last week (with Julius Berner, Jingtong (Jeff) Sun). You can find the recording here: youtube.com/watch?v=ue8liZ…
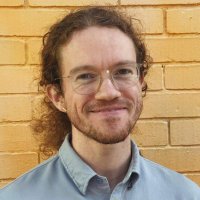
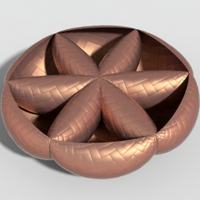
Need to solve PDEs, and struggle with meshing? Heard about "Walk on Spheres," but didn't know where to start? Check out the awesome intro course by Rohan Sawhney and Bailey Miller, just posted from #SGP2024: youtube.com/watch?v=1u-5b4…
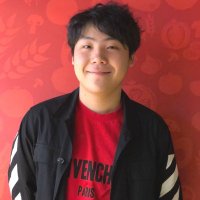
If you are traveling to ICML Conference 2024 next week, don't miss our workshop on structured prob. inference and generative modeling on Friday in room Lehar 3! We have a stellar list of speakers to share the frontiers of sampling, Bayesian inference, generative models, and beyond!
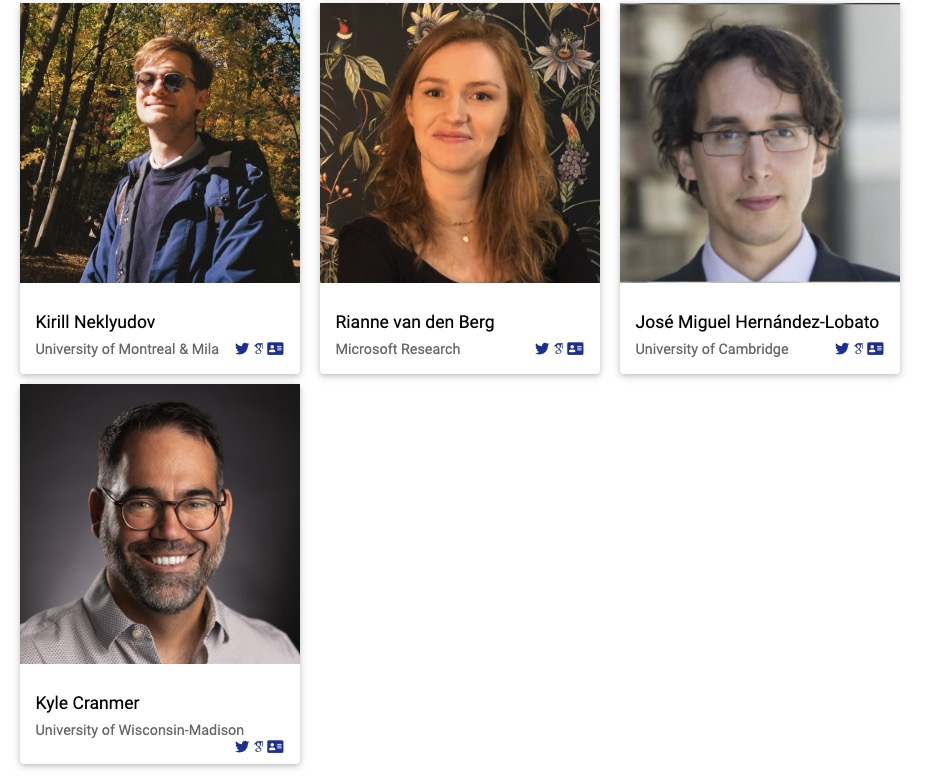
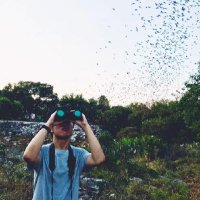
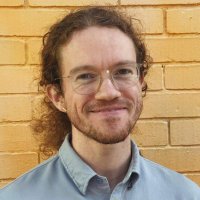
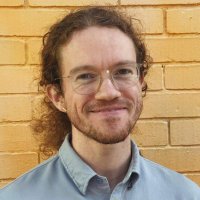
2a. Deep Generalized Schrödinger Bridge by Guan-Horng Liu Tianrong Chen 陈天荣 Oswin So E. Theodorou 2b. DGSB Matching by Guan-Horng Liu Yaron Lipman Maximilian Nickel B. Karrer E. Theodorou Ricky T. Q. Chen These also add population-level Lagrangians to SB x.com/guanhorng_liu/…
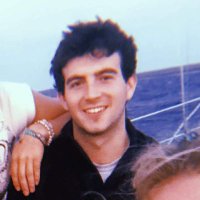
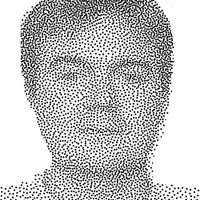
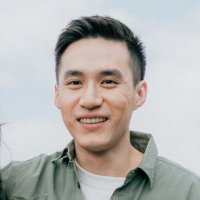
📢 Life update: I've joined AI at Meta (#FAIR #NYC) as #ResearchScientist this week 🍎! Extremely grateful for everyone who's supported me along the way 🙂 I'll keep working on flow/diffusion for structural problems, Schrodinger bridges, optimization, stochastic control, & more🏃🏻♂️