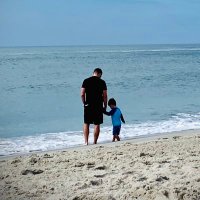
Edward Kennedy
@edwardhkennedy
assoc prof of statistics & data science @CMU_Stats @CarnegieMellon. interested in causality, machine learning, nonparametrics, criminal justice, public policy
ID: 1012125662117851136
http://www.ehkennedy.com 28-06-2018 00:08:57
1,1K Tweet
4,4K Followers
592 Following
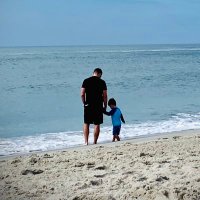
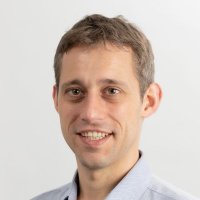
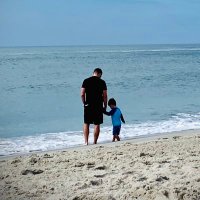
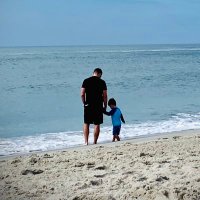
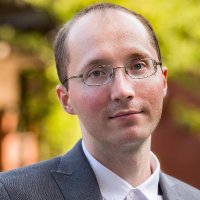
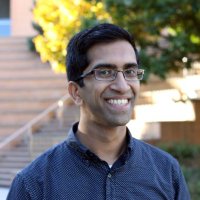
"Position: Considerations for Differentially Private Learning with Large-Scale Public Pretraining," with Florian Tramèr & Nicholas Carlini got an #ICML2024 best paper award! x.com/thegautamkamat… 🧵: the personal side of this research, emotional high & lows, & more 👇 1/n
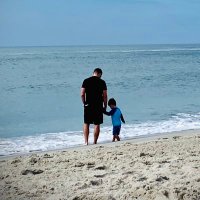
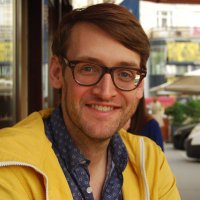
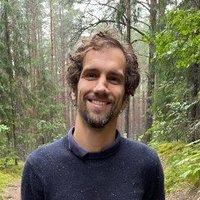
Amazing presentations by Susan Athey and guido imbens Designing Complex Experiments youtube.com/watch?v=I6GyDW…
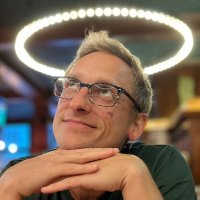
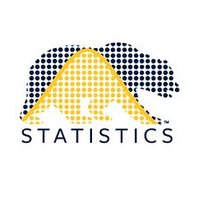
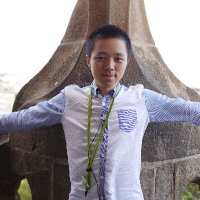
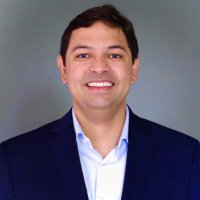
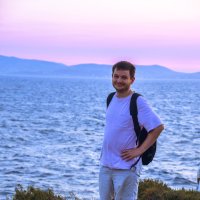
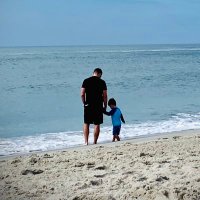