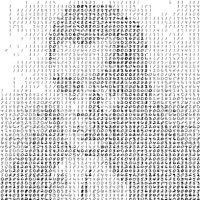
Griffiths Computational Cognitive Science Lab
@cocosci_lab
Tom Griffiths' Computational Cognitive Science Lab. Studying the computational problems human minds have to solve.
ID: 1291487042921168898
http://cocosci.princeton.edu/ 06-08-2020 21:31:29
144 Tweet
4,4K Followers
131 Following
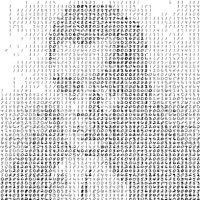
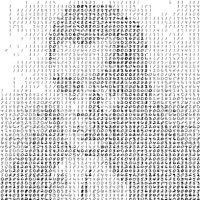
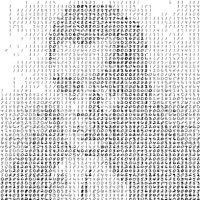
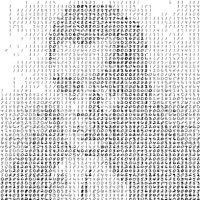
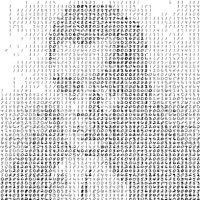
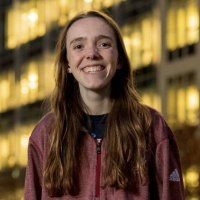
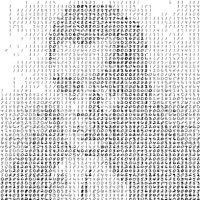
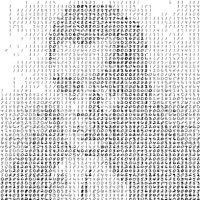

My latest paper is out at Psych Sci❗️ w/ Tania Lombrozo & Griffiths Computational Cognitive Science Lab We built a Bayesian model that captures people's inferences from opinions* in a game-show paradigm. *e.g., if 12 people think 'X' is True, and 2 think 'X' is False, P(X=T) = 81% journals.sagepub.com/doi/10.1177/09…
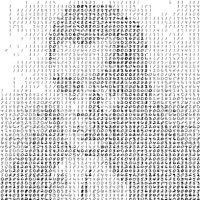
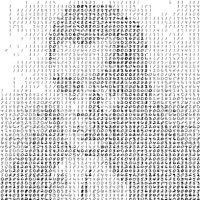
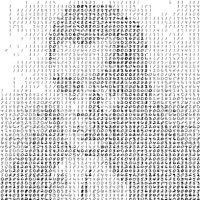