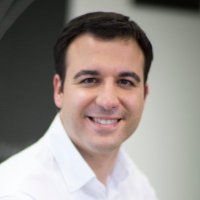
Jorge Bravo
@bravo_abad
Prof. of Physics @UAM_Madrid | Profesor Titular. PI of the AI for Materials Lab | Director del Laboratorio de IA para Materiales.
ID: 1976371410
https://www.ai4materials.org/ 20-10-2013 21:02:11
2,2K Tweet
5,5K Takipçi
4,4K Takip Edilen