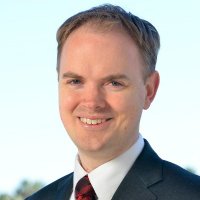
Mykel Kochenderfer
@aiprof_mykel
ID: 1321177724229054465
https://mykel.kochenderfer.com 27-10-2020 19:52:04
53 Tweet
418 Takipçi
4 Takip Edilen
@aiprof_mykel
ID: 1321177724229054465
https://mykel.kochenderfer.com 27-10-2020 19:52:04
53 Tweet
418 Takipçi
4 Takip Edilen