
carlos
@_carlosejimenez
phd student @princeton_nlp.
Message me on substack.com/@closji
ID: 1124834159841562624
https://www.carlosejimenez.com/ 05-05-2019 00:32:16
196 Tweet
982 Followers
489 Following
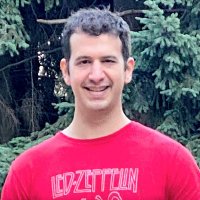
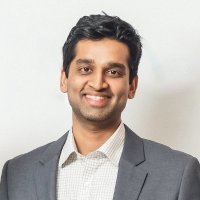
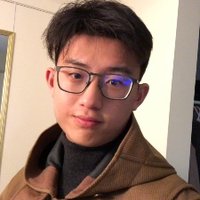
Excited to share what I did Sierra with Noah Shinn pedram and Karthik Narasimhan ! 𝜏-bench evaluates critical agent capabilities omitted by current benchmarks: robustness, complex rule following, and human interaction skills. Try it out!
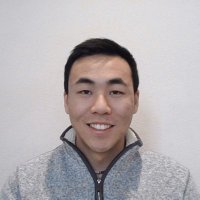
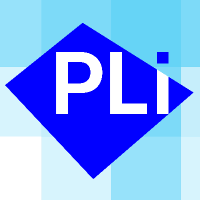
Don't miss our upcoming seminar this Thursday, 6/27, at 3 pm EST on Zoom Ofir Press will discuss the autonomous system SWE-agent, as well as SWE-bench, the benchmark for measuring performance. Register now: lu.ma/az1hdsa4
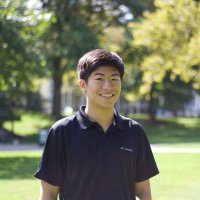
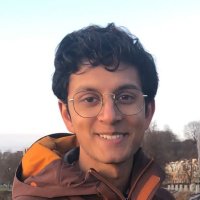
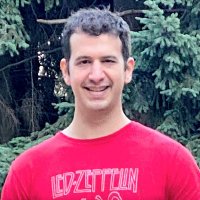
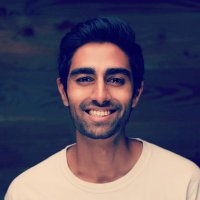
The GenAI Collective had the privilege of hosting the esteemed Princeton researchers behind SWE-bench and SWE-agent at our first ever NYC research meetup! Huge shoutout to Ofir Press John Yang carlos and Kilian Lieret for informative talks and hanging with our community The
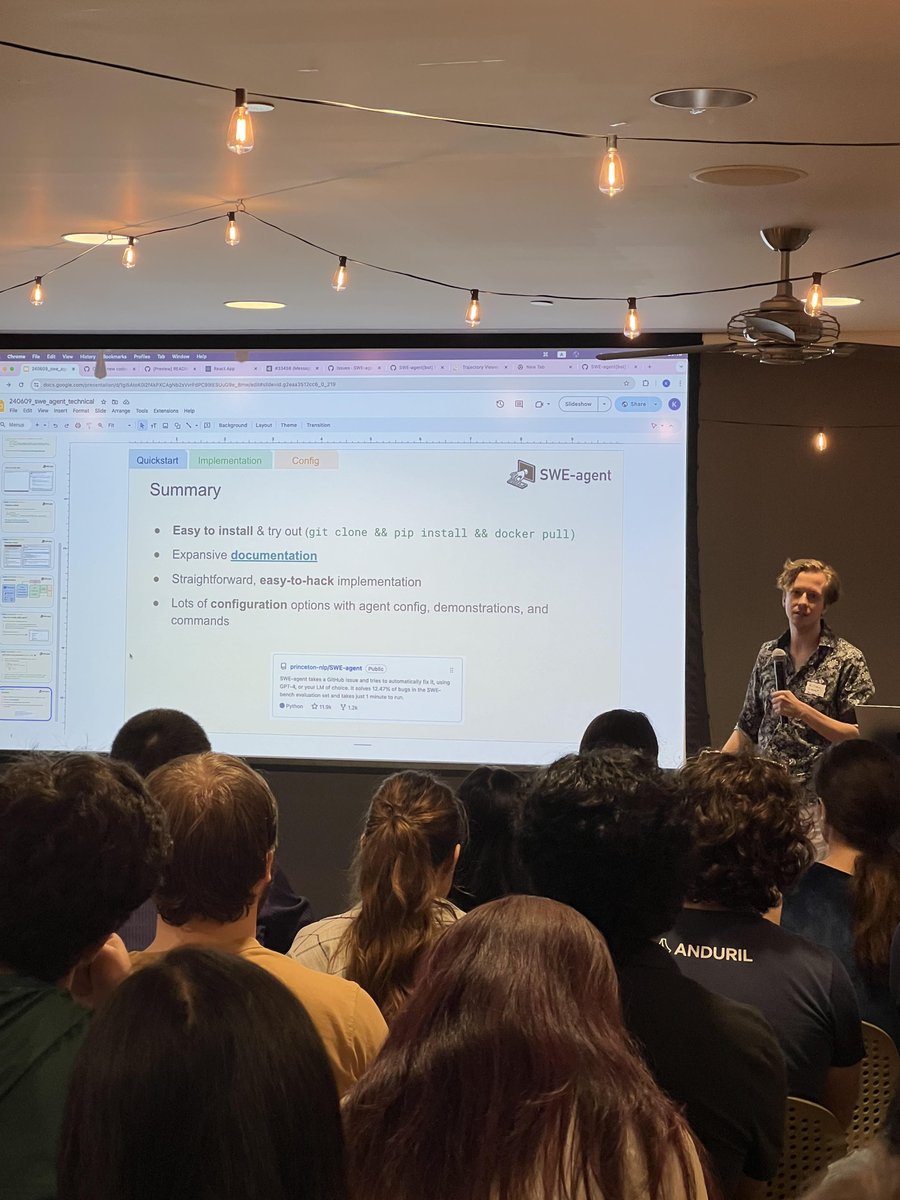
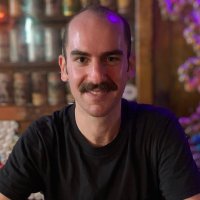
How can we understand neural chatbots in terms of interpretable, symbolic mechanisms? To explore this question, we constructed a Transformer that implements the classic ELIZA chatbot algorithm (with Abhishek Panigrahi and Danqi Chen). Paper: arxiv.org/abs/2407.10949 (1/6)
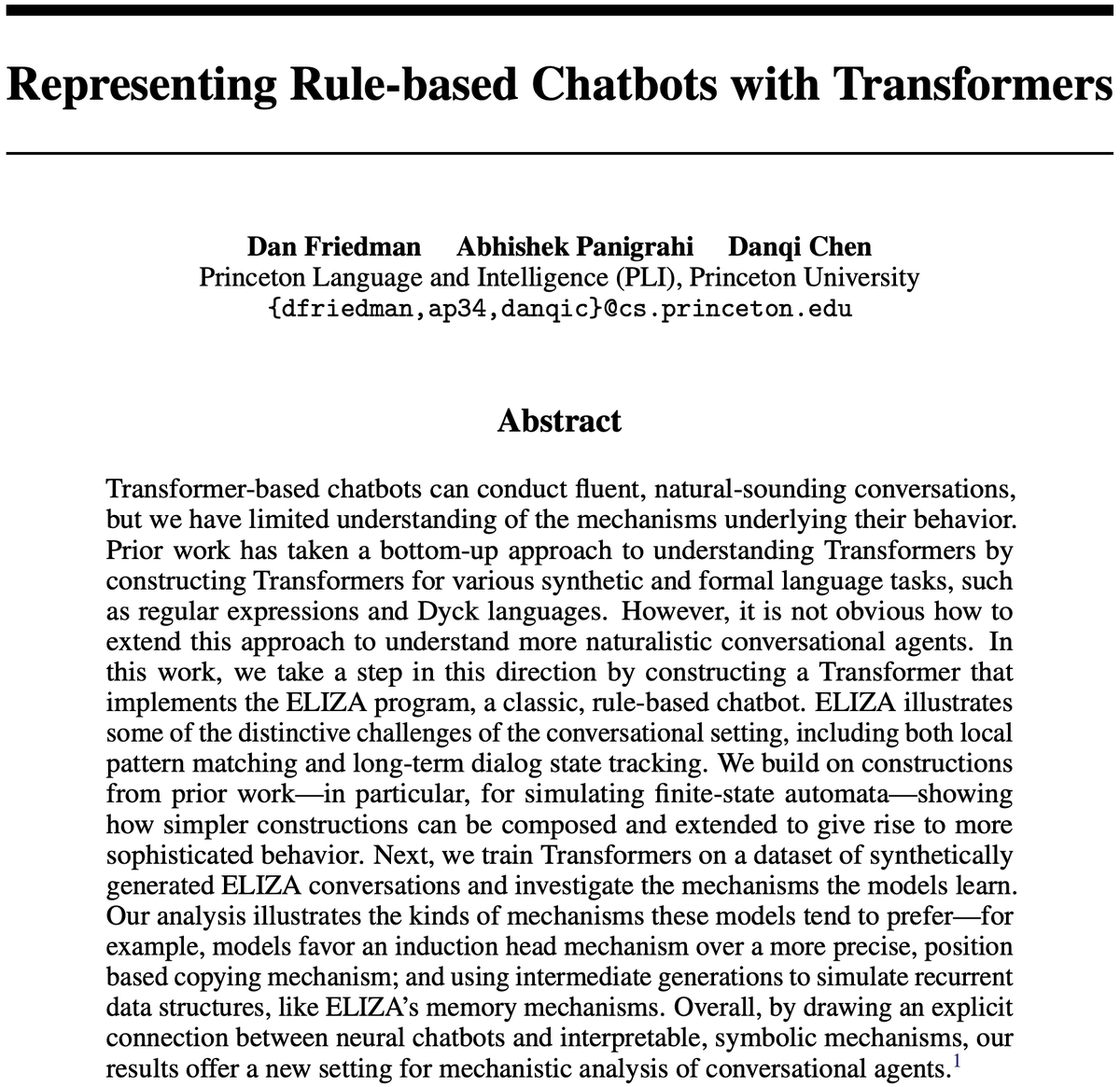
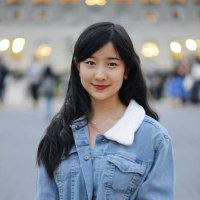
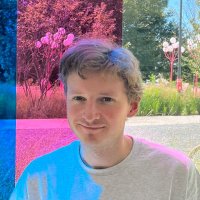
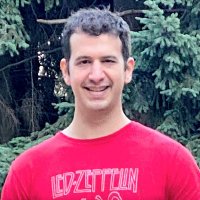
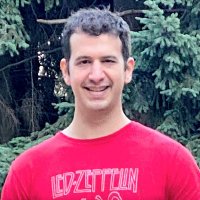

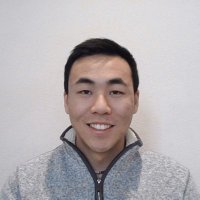
