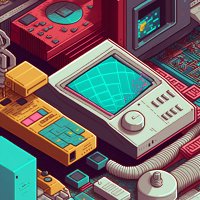
Ethan Mollick
@emollick
Professor @Wharton studying AI, innovation & startups. Democratizing education using tech
Book: https://t.co/CSmipbJ2jV
Substack: https://t.co/UIBhxu4bgq
ID:39125788
https://mgmt.wharton.upenn.edu/profile/emollick/ 10-05-2009 22:33:52
26,4K Tweets
209,8K Followers
551 Following